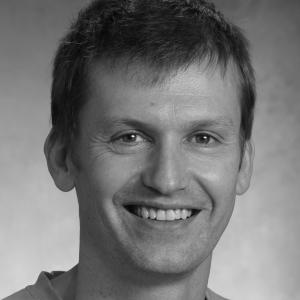
Member of the Center for Cognitive Neuroscience
Faculty Network Member of the Duke Institute for Brain Sciences
Email: nicolas.brunel@duke.edu
Brunel Lab
We use theoretical models of brain systems to investigate how they process and learn information from their inputs. Our current work focuses on the mechanisms of learning and memory, from the synapse to the network level, in collaboration with various experimental groups.
Our research has spanned five major directions:
- Dynamics of networks of spiking neurons: What are the mechanisms of the low-rate, highly irregular activity that is ubiquitous in cerebral cortex? To answer this question, we have introduced models of networks of integrate-and-fire neurons composed of two populations of neurons (excitatory and inhibitory) with random connectivity, and systematically analyzed their dynamics.
- Stochastic dynamics of single spiking neurons: To understand better the factors that shape the neuronal response to transient inputs, we have investigated mathematically the dynamics of the instantaneous firing probability in single spiking neuron models of increasing complexity in the presence of noisy inputs. We have characterized extensively how the instantaneous firing rate depends on the statistics of the inputs, in both static (f-I curve) and dynamic (response to transient inputs) conditions.
- Mechanisms of persistent activity in cortical circuits: Selective persistent activity has been hypothesized to be the substrate of working memory in cortical circuits during delayed response tasks in behaving primates. We have built network models of spiking neurons that are able to reproduce the phenomenology of these experiments.
- Synaptic plasticity rules: What are the rules of synaptic plasticity? We have followed three complementary approaches to make progress on this question: (i) building a synaptic plasticity model from in vitro data (ii) inferring plasticity rules from in vivo data (iii) deriving learning rules from optimality principles.
- Statistics of networks optimizing information storage: What are the consequences of optimizing the amount of stored information, or its robustness, on the structure and statistics of neuronal connectivity? We have computed analytically the statistics of synaptic connectivity in a network that maximizes the amount of stored information, with a given robustness constraint so the information can be retrieved robustly in the presence of noise, and shown than the resulting statistics are in good agreement with in vitro cortical data.
Selected publications
N Brunel (2016), Is cortical connectivity optimized for storing information?, Nature Neurosci., 19:749-755
S Lim, J McKee, L Woloszyn, Y Amit, D Freedman, D Sheinberg and N Brunel (2015), Inferring learning rules from distributions of firing rates in cortical neurons, Nature Neurosci., 18:1804-1810
M Graupner and N Brunel (2012), A calcium-based plasticity model explains sensitivity of synaptic changes to spike pattern, rate and dendritic location, Proc Natl Acad Sci U S A., 109:3991-3996
N Brunel, V Hakim, P Isope, JP Nadal and B Barbour (2004), Optimal information storage and the distribution of synaptic weights: Perceptron vs. Purkinje cell, Neuron, 43:745-757
N Fourcaud-Trocme, D Hansel, C van Vreeswijk and N Brunel (2003), How spike generation mechanisms determine the neuronal response to fluctuating inputs, J. Neurosci., 23:11628-11640
N Brunel, F Chance, N Fourcaud and L Abbott (2001), Effects of synaptic noise and filtering on the frequency response of spiking neurons, Phys. Rev. Lett., 86:2186-2189
N Brunel (2000), Dynamics of sparsely connected networks of excitatory and inhibitory spiking neurons, J. Comp. Neurosci 8:183-208
N Brunel and V Hakim (1999), Fast global oscillations in networks of integrate-and-fire neurons with low firing rates, Neural Comp., 11:1621-1671
DJ Amit and N Brunel (1997), Model of global spontaneous activity and local structured activity during delay periods in the cerebral cortex, Cereb.~Cortex, 7:237-252
Brunel Research
Dynamics of networks of spiking neurons
We have introduced models of networks of integrate-and-fire neurons composed of two populations of neurons (excitatory and inhibitory) with random connectivity, and analyzed their dynamics [11,13,20]. We used an analytical framework in which each population is described by its distribution of membrane potentials, as well as its instantaneous firing probability [19,20]. A crucial feature of this formalism is to take into account the temporal fluctuations in the inputs received by neurons, that are essential to capture the phenomenology of electrophysiological recordings in cortex (highly irregular and low rate activity). In network states that capture this data, mean inputs to neurons are well below the firing threshold, and firing is induced by fluctuations around these mean inputs (the so-called fluctuation-driven regime). The formalism can also account for the emergence of synchronous population oscillations in such networks [19,20]. In the inhibition dominated regime, these oscillations tend to be fast (frequencies from the gamma to the ultra fast range). The analysis identified a regime in which synchronous population oscillations coexist with highly irregular firing of single neurons at rates that are much lower than the population oscillation. In vivo data during oscillatory episodes in cortex and hippocampus support this stochastic oscillatory model, since firing rates are typically lower than the population frequency.
Stochastic dynamics of single spiking neurons
To understand the factors that shape the neuronal response to transient inputs, we have investigated the dynamics of the instantaneous firing probability in single spiking neuron models of increasing complexity in the presence of noisy inputs [23,25,26,28,29,31,34,52, 60,64]. We have characterized extensively how the instantaneous firing rate depends on the statistics of the inputs, in both static and dynamic conditions. Along the way, several simplified models whose complexity is intermediate between the simple leaky integrate-and-fire model and Hodgkin-Huxley (HH) type models have been introduced. In particular, we introduced a simplified non-linear one variable model (the exponential integrate-and-fire (EIF) model) that describes accurately the spike generation dynamics of HH type models [31]. This model allowed us to understand the factors that limit the speed of the neuronal response to fast transient inputs. The EIF model has been shown subsequently to fit well the dynamics of action potential generation in both cortical pyramidal cells and fast-spiking interneurons, and it forms the basis of adaptive EIF models, that are increasingly used in many network models, since they combine simplicity, ability to fit quantitatively electrophysiological data, and ability to produce a large diversity of electrophysiological behaviors. We have also shown that a population of EIF neurons can be reduced mathematically in the strong noise regime to a firing rate model [52].
Mechanisms of persistent activity in cortical circuits
In parallel with the study of the dynamics of unstructured networks, we have investigated the dynamics of networks that are stuctured by learning, with the aim to understand better phenomena observed in cortex of behaving primates during delayed response tasks, such as selective persistent activity [11,13,21,22,24]. Selective persistent activity has been hypothesized to be the substrate of working memory in cortical circuits. We have built network models of spiking neurons in which persistent activity is due to multi-stability of the network, which arises thanks to a combination of potentiated connectivity between selective sub-groups of pyramidal cells, and strong global inhibition. We also studied how temporal associations between stimuli can be learned in such networks, and how association learning can lead to the phenomenon of prospective activity (increase in activity in neurons that are selective to stimuli that the animal expects to occur after the delay period) [30].
Synaptic plasticity rules
We have followed three complementary approaches to make progress on our understanding of synaptic plasticity. The first approach consists in building a synaptic plasticity model from in vitro data. We introduced a simplified synaptic plasticity model based on post-synaptic calcium concentration [55], that can reproduce the diversity of spike-timing dependent plasticity (STDP) curves (how synaptic efficacy changes as a function of timing difference between pre and post-synaptic neurons) seen in experiments, varying only two parameters characterizing the amplitude of calcium transients induced by pre and post-synaptic spikes. The second approach consists in inferring plasticity rules from in vivo data. We have been using electrophysiological data from behaving monkeys (visual responses of IT neurons to large sets of novel and highly familiar stimuli). We introduced a method that allowed us to infer directly the learning rule (or more precisely, the dependence of the rule on post-synaptic firing rate) that produces the observed changes in the response statistics, from the corresponding two distributions of visual responses (to novel and familiar stimuli, respectively) [66]. The third and last approach is to derive learning rules from optimality principles. I have derived plasticity rules that can approach the maximal storage capacity in several contexts [38,65].
Statistics of networks optimizing information storage
What are the consequences of optimizing the amount of stored information, or its robustness, on the statistics of neuronal connectivity? We computed analytically the distribution of synaptic weights in a network that maximizes the amount of stored information, with a given robustness constraint so the information can be retrieved robustly in the presence of noise. When information is stored in excitatory synapses, we showed that the distribution contains a finite and large fraction of exactly zero synaptic weights, which can be interpreted either as `silent’ synapses or `potential’ synapses The fraction of `silent’ or `potential’ synapses can be shown to be always larger than 0.5, and increases with the degree of robustness of information storage. We also showed that the distribution of synaptic weights can fit observed distribution of weights in Purkinje cells and cortical pyramidal cells [32]. We have computed the joint distributions of pairs of synaptic weights in recurrent networks that maximize the numbers of fixed point attractors, or the number of stored sequences of activity [69]. Networks that maximize the numbers of fixed point attractors have a strong over-representation of reciprocally connected pairs of excitatory neurons, in agreement with data from several multiple intracellular recording studies in cortical slices, while networks that maximize the numbers of stored sequences of activity have no such over-representation.
Brunel Lab Members
Brunel Publications
Aljadeff, Johnatan, Maxwell Gillett, Ulises Pereira Obilinovic, and Nicolas Brunel. “From synapse to network: models of information storage and retrieval in neural circuits.” Current Opinion in Neurobiology 70 (October 2021): 24–33. https://doi.org/10.1016/j.conb.2021.05.005.
Inglebert, Yanis, Johnatan Aljadeff, Nicolas Brunel, and Dominique Debanne. “Synaptic plasticity rules with physiological calcium levels.” Proc Natl Acad Sci U S A 117, no. 52 (December 29, 2020): 33639–48. https://doi.org/10.1073/pnas.2013663117.
Gillett, Maxwell, Ulises Pereira, and Nicolas Brunel. “Characteristics of sequential activity in networks with temporally asymmetric Hebbian learning.” Proc Natl Acad Sci U S A 117, no. 47 (November 24, 2020): 29948–58. https://doi.org/10.1073/pnas.1918674117.
Sanzeni, Alessandro, Mark H. Histed, and Nicolas Brunel. “Response nonlinearities in networks of spiking neurons.” Plos Comput Biol 16, no. 9 (September 2020): e1008165. https://doi.org/10.1371/journal.pcbi.1008165.
Sanzeni, Alessandro, Bradley Akitake, Hannah C. Goldbach, Caitlin E. Leedy, Nicolas Brunel, and Mark H. Histed. “Inhibition stabilization is a widespread property of cortical networks.” Elife 9 (June 29, 2020). https://doi.org/10.7554/eLife.54875.
Fore, Taylor R., Benjamin N. Taylor, Nicolas Brunel, and Court Hull. “Acetylcholine Modulates Cerebellar Granule Cell Spiking by Regulating the Balance of Synaptic Excitation and Inhibition.” J Neurosci 40, no. 14 (April 1, 2020): 2882–94. https://doi.org/10.1523/JNEUROSCI.2148-19.2020.
Oleskiw, Timothy D., Wyeth Bair, Eric Shea-Brown, and Nicolas Brunel. “Firing rate of the leaky integrate-and-fire neuron with stochastic conductance-based synaptic inputs with short decay times,” February 25, 2020.
Vaz, Alex P., Sara K. Inati, Nicolas Brunel, and Kareem A. Zaghloul. “Coupled ripple oscillations between the medial temporal lobe and neocortex retrieve human memory.” Science (New York, N.Y.) 363, no. 6430 (March 2019): 975–78. https://doi.org/10.1126/science.aau8956.
Pereira, Ulises, and Nicolas Brunel. “Unsupervised Learning of Persistent and Sequential Activity.” Frontiers in Computational Neuroscience 13 (January 2019): 97. https://doi.org/10.3389/fncom.2019.00097.
Bouvier, Guy, Johnatan Aljadeff, Claudia Clopath, Célian Bimbard, Jonas Ranft, Antonin Blot, Jean-Pierre Nadal, Nicolas Brunel, Vincent Hakim, and Boris Barbour. “Cerebellar learning using perturbations.” Elife 7 (November 12, 2018). https://doi.org/10.7554/eLife.31599.