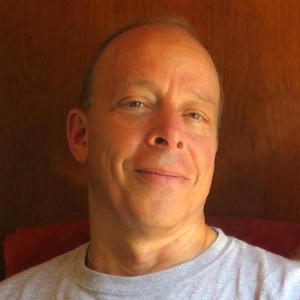
Faculty Network Member of the Duke Institute for Brain Sciences
Duke University E-mail: hsg@phy.duke.edu
Science Drive Office phone: 919-660-2548
Box 90305 Fax: 919-660-2525
Durham , North Carolina, USA 27708-0305
Greenside Lab
Welcome to the home page of my research group in the Physics Department at Duke University. My group works on a variety of topics related to sustained nonequilibrium physical systems, including spatiotemporal chaos and the modeling and simulation of birdsong.
An example of the kind of question that interests my group is understanding why the weather is hard to forecast. Most people take for granted that the weather is unpredictable but what precisely makes it so? Is it because the radius of the earth is large compared to the depth of the atmosphere, so that the atmosphere consists of many uncorrelated regions of dynamical activity? Is it because the atmosphere is strongly driven out of equilibrium and so is turbulent? On what details does the forecasting time of about two weeks actually depend?
A similar question of interest is how fibrillation commences in a mammalian heart, i.e., how does the usual coherent periodic beating change to an incoherent nonperiodic dynamics? As one clue, the occurrence of fibrillation is known to be size-dependent, e.g., animals with small hearts such as mice or guinea pigs do not have heart attacks and fibrillation, if induced artificially in such hearts, spontaneously returns to a periodic dynamics. Why are big hearts more susceptible to fibrillation? Do whales, whose hearts can approach six feet in diameter, have heart attacks and, if not, how is fibrillation avoided? More generally, how does the dynamics of any nonequilibrium system depend on its size?
Rather than study such difficult questions directly, my group follows a theoretical physics tradition of finding simple idealized mathematical models that contain essential mechanisms of complex dynamics. We then study these models, usually by computer simulation, to answer some basic questions: what kinds of transitions (bifurcations) lead from simple to more complex dynamics? What kinds of spatiotemporal states are possible and how do they depend on parameters? How does the transport of energy and mass depend on the spatiotemporal structure of some medium?
A related part of my group's research is finding new or better ways to characterize the spatiotemporal disorder found in many nonequilibrium systems. What is really meant by the word "complex" and how does one compare one complex state with another, e.g., a computer simulation with experimental data?
Often our effort is directed towards understanding simpler experimental systems such as the dynamics of a shallow wide layer of convecting fluid (Rayleigh-Benard convection) since such experimental systems are much more completely characterized than the weather or a heart. E.g., scientists can perform convection experiments in which essentially all details are known and controlled to a high accuracy and for which we have high confidence that a complete quantitative description is available (the Boussinesq equations). This allows one to test quantitatively theory against experiment with an accuracy and confidence that is not possible in the fields of meteorology or physiology. Such quantitative comparisions of theory with experiment are essential if broad fundamental principles of nonequilibrium dynamics are to be discovered.
In carrying out this research, my group is grateful for support from the National Science Foundation and the Department of Energy.
Greenside Research
Birdsong: My group is currently collaborating with Richard Mooney's birdsong group to understand the neural circuitry of auditory-guided vocal learning in songbirds, since this is a promising experimental paradigm for investigating the neural basis of human speech. Of especial interest is to understand how the nuclei of the premotor pathway (HVc and RA) and of the anterior forebrain pathway (X to DLM to lMAN to X) coordinate their activities so that young songbirds can learn the adult songs appropriate to their species.
Olfaction: A few years ago, I spent a sabbatical year in the laboratory of Dr. Larry Katz, whose group carries out research on mammalian olfaction. Key questions are how do the spatiotemporal electrochemical patterns of the main olfactory bulb and of the accessory olfactory bulb enable an animal to classify odors, to remember odors, and to identify certain mixtures of odors (e.g., pheromone) as a unique perception.
EEG data analysis: I have collaborated with Dr. Andrew Krystal of Duke University's Medical Center on applications of nonlinear dynamics to the analysis of EEG data derived in the context of helping severely depressed patients by electorconvulsive therapy (ECT). While the longer-term clinical goal is to find ways to improve the efficacy of ECT treatments, a shorter term research goal was to understand how to take into account the strongly nonstationary character of a given EEG recording and how to detect and take into account the substantial statistical variability of EEG recordings, even when data from one patient is compared with data of the same patient a week later. Andrew and I are also interested in some of the questions that fascinate many other people about EEG data, e.g,, how much cognitive and clinical information can be extracted from an EEG, which represents a coarse averaging of signals from millions of cortical neurons?
Greenside Publications
McCreery, K., and H. Greenside. “The electric field of a uniformly charged cubic shell.” American Journal of Physics 86, no. 1 (January 1, 2018): 36–44. https://doi.org/10.1119/1.5009446.
Jackson, David P. “AJP Reviewers.” American Journal of Physics 84, no. 12 (December 2016): 901–2. https://doi.org/10.1119/1.4966631.
Lim, M. X., and H. Greenside. “The external magnetic field created by the superposition of identical parallel finite solenoids.” American Journal of Physics 84, no. 8 (August 1, 2016): 606–15. https://doi.org/10.1119/1.4948603.
Cross, M., and H. Greenside. Pattern formation and dynamics in nonequilibrium systems, 2009. https://doi.org/10.1017/CBO9780511627200.
Li, MengRu, and Henry Greenside. “Stable propagation of a burst through a one-dimensional homogeneous excitatory chain model of songbird nucleus HVC.” Physical Review. E, Statistical, Nonlinear, and Soft Matter Physics 74, no. 1 Pt 1 (July 26, 2006): 011918. https://doi.org/10.1103/physreve.74.011918.
Jayaraman, A., J. D. Scheel, H. S. Greenside, and P. F. Fischer. “Characterization of the domain chaos convection state by the largest Lyapunov exponent.” Physical Review. E, Statistical, Nonlinear, and Soft Matter Physics 74, no. 1 Pt 2 (July 26, 2006): 016209. https://doi.org/10.1103/physreve.74.016209.
Chiam, K. H., M. C. Cross, H. S. Greenside, and P. F. Fischer. “Enhanced tracer transport by the spiral defect chaos state of a convecting fluid.” Physical Review. E, Statistical, Nonlinear, and Soft Matter Physics 71, no. 3 Pt 2A (March 11, 2005): 036205. https://doi.org/10.1103/physreve.71.036205.
Paul, M. R., K. H. Chiam, M. C. Cross, P. F. Fischer, and H. S. Greenside. “Pattern formation and dynamics in Rayleigh-Bénard convection: Numerical simulations of experimentally realistic geometries.” Physica D: Nonlinear Phenomena 184, no. 1–4 (October 1, 2003): 114–26. https://doi.org/10.1016/S0167-2789(03)00216-1.
Cherry, Elizabeth M., Henry S. Greenside, and Craig S. Henriquez. “Efficient simulation of three-dimensional anisotropic cardiac tissue using an adaptive mesh refinement method.” Chaos (Woodbury, N.Y.) 13, no. 3 (September 2003): 853–65. https://doi.org/10.1063/1.1594685.
Chiam, K. -. H., Ming-Chih Lai, and Henry S. Greenside. “Efficient algorithm on a nonstaggered mesh for simulating Rayleigh-Bénard convection in a box.” Physical Review. E, Statistical, Nonlinear, and Soft Matter Physics 68, no. 2 Pt 2 (August 29, 2003): 026705. https://doi.org/10.1103/physreve.68.026705.